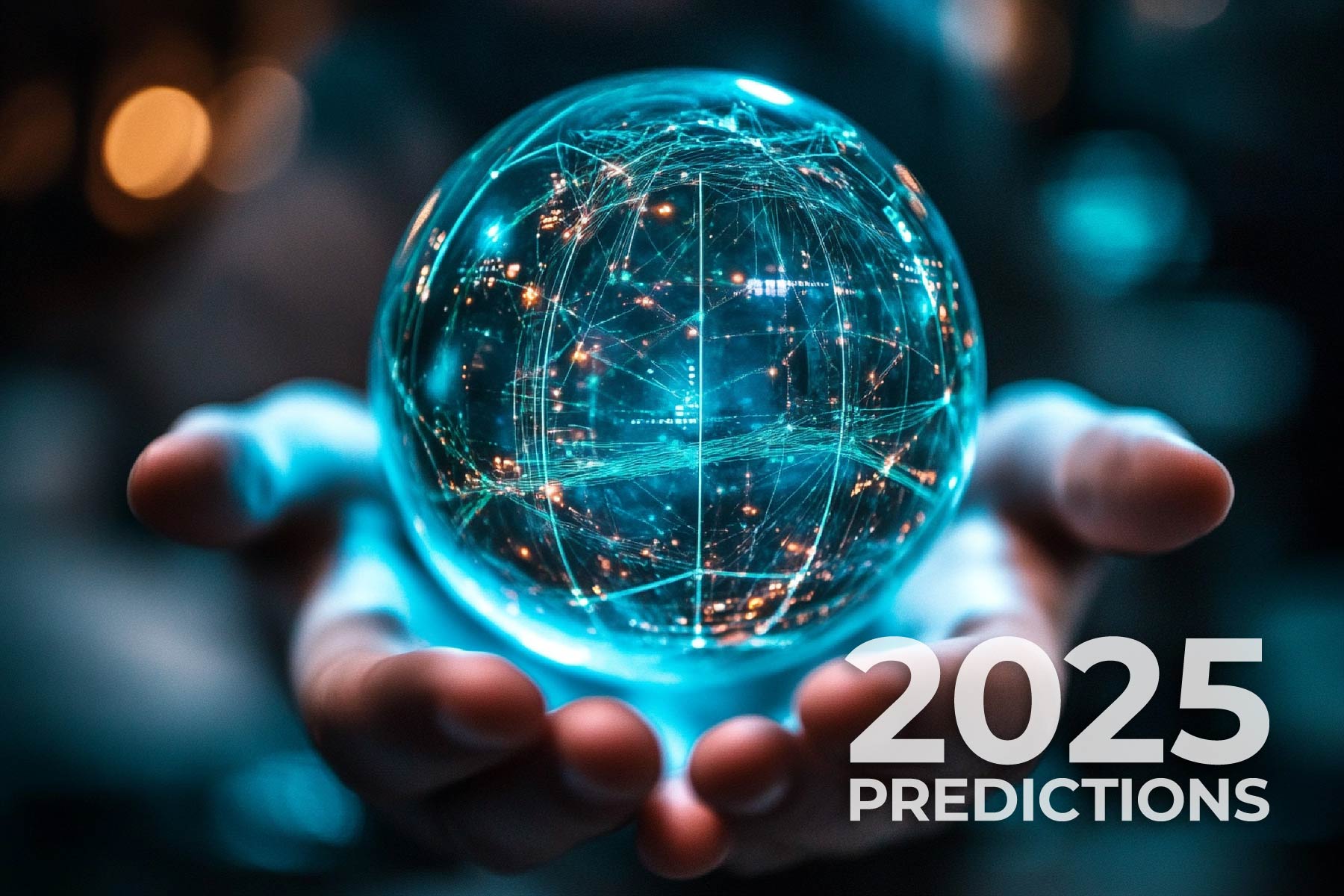
Bob Rogers’ 5 AI Predictions for 2025
As 2024 draws to a close, I began to reflect both on the year behind us and the transformative changes ahead. Below are my five major predictions for AI in 2025.
Prediction 1: AI is going to move past simply using previous linguistic patterns to generate text and will begin to use a framework for associating facts and knowledge with linguistic patterns. Today, AI talks a good game, but doesn’t know anything. I predict that this will change in 2025, probably as the result of a marriage between knowledge graphs and LLMs. It will require a new architecture to make it work, so it's not as simple as combining knowledge graphs and LLM in a graphRAG type of application.
Prediction 2: 2025 will be the year of the Chief AI Officer. Enterprises large and small will hire someone responsible for ensuring that AI projects make sense, that security and regulatory structures are in place, and that use guidelines are published and enforced. Chief AI Officers will be responsible for minimizing the risk of organizational damage caused by hallucination and will need to ensure that enterprises are not investing in products that don't actually work. I recently heard a Chief AI Officer say that they are looking to block “fly-by-night” AI products.
Prediction 3: We will see a proliferation of locally-deployed small GenAI models along the lines of Llama 3 that can be tuned or pre-prompted for very specific use cases. These applications will be especially important for use cases that require access to highly sensitive data or secure mobility. We are seeing an increasing number of “LLMOps” products that address the need to seamlessly train, test, deploy, and update such local models. Infrastructure costs to deploy local models have come down, making the economics of local models more sensible, but the risk is that without an integrated LLMOps pipeline, locally deployed models could become hopelessly out of date every few months.
Prediction 4: Enterprises will move beyond simple AI guidelines to begin to deploy real regulatory and governance frameworks and tooling that allows them to monitor model performance, data quality, regulatory compliance, security, and network connectivity. Many of these monitoring activities on their AI infrastructure will be done by agents.
Prediction 5: Agentic AI, which I view as using an AI quarterback to help automatically pick which specific AI tool or service is best for each enterprise application, will begin to become more commonplace. This is a natural evolution of the current practice of using ChatGPT, Claude, Gemini, Co-pilot and other tools to generate content and solve problems, and then comparing the quality and validity of each before choosing which output to use.
As 2024 draws to a close, I began to reflect both on the year behind us and the transformative changes ahead. Below are my five major predictions for AI in 2025.
Prediction 1: AI is going to move past simply using previous linguistic patterns to generate text and will begin to use a framework for associating facts and knowledge with linguistic patterns. Today, AI talks a good game, but doesn’t know anything. I predict that this will change in 2025, probably as the result of a marriage between knowledge graphs and LLMs. It will require a new architecture to make it work, so it's not as simple as combining knowledge graphs and LLM in a graphRAG type of application.
Prediction 2: 2025 will be the year of the Chief AI Officer. Enterprises large and small will hire someone responsible for ensuring that AI projects make sense, that security and regulatory structures are in place, and that use guidelines are published and enforced. Chief AI Officers will be responsible for minimizing the risk of organizational damage caused by hallucination and will need to ensure that enterprises are not investing in products that don't actually work. I recently heard a Chief AI Officer say that they are looking to block “fly-by-night” AI products.
Prediction 3: We will see a proliferation of locally-deployed small GenAI models along the lines of Llama 3 that can be tuned or pre-prompted for very specific use cases. These applications will be especially important for use cases that require access to highly sensitive data or secure mobility. We are seeing an increasing number of “LLMOps” products that address the need to seamlessly train, test, deploy, and update such local models. Infrastructure costs to deploy local models have come down, making the economics of local models more sensible, but the risk is that without an integrated LLMOps pipeline, locally deployed models could become hopelessly out of date every few months.
Prediction 4: Enterprises will move beyond simple AI guidelines to begin to deploy real regulatory and governance frameworks and tooling that allows them to monitor model performance, data quality, regulatory compliance, security, and network connectivity. Many of these monitoring activities on their AI infrastructure will be done by agents.
Prediction 5: Agentic AI, which I view as using an AI quarterback to help automatically pick which specific AI tool or service is best for each enterprise application, will begin to become more commonplace. This is a natural evolution of the current practice of using ChatGPT, Claude, Gemini, Co-pilot and other tools to generate content and solve problems, and then comparing the quality and validity of each before choosing which output to use.