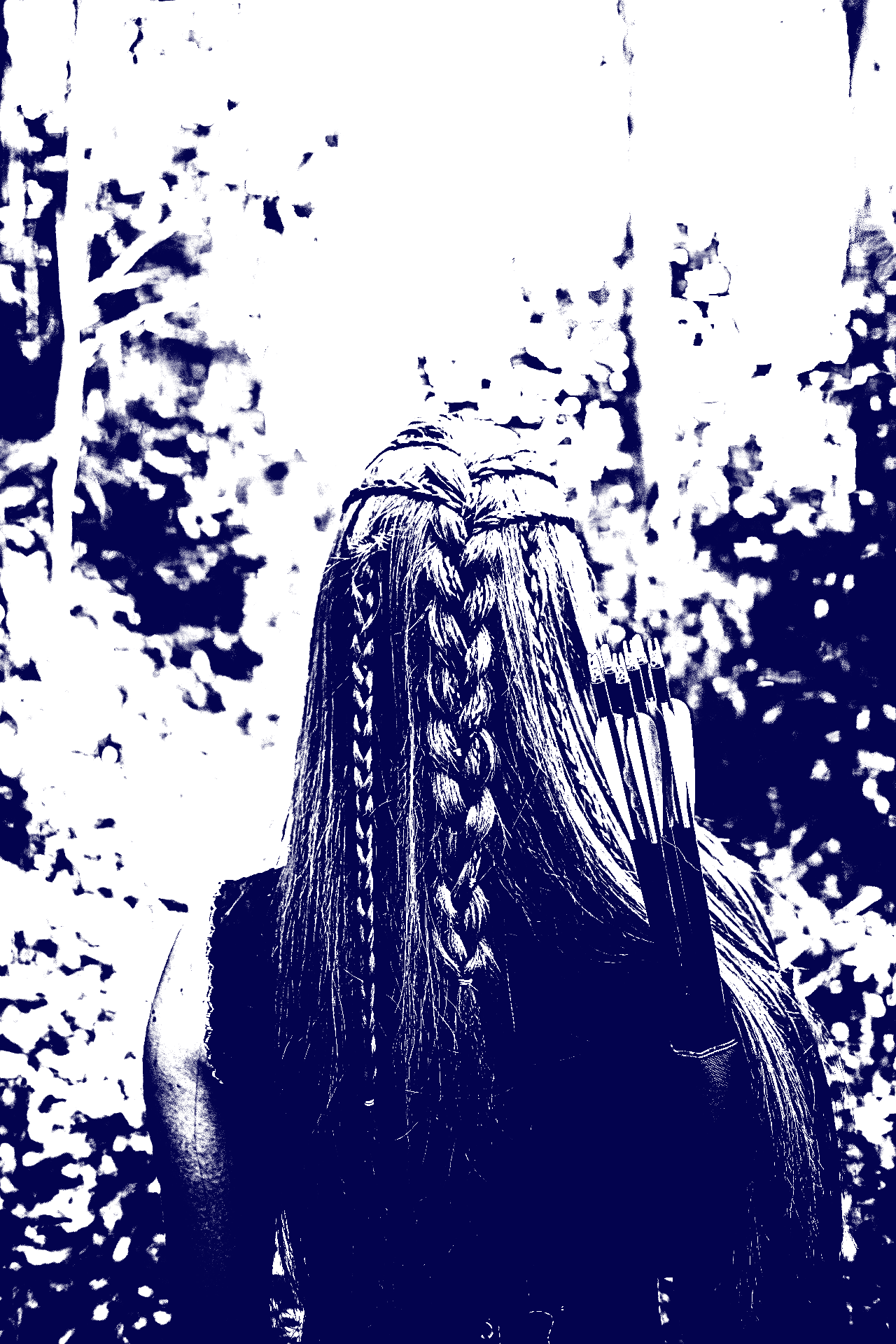
Lemurian Labs: A Robin Hood for AI Access
One thing you notice when you talk to Jay Dawani is that when he sees a challenge that’s important enough, he actually takes it on himself to solve it. This was made clear when Jay delivered the how to for coding AI algorithms based on a foundation of high school math to democratize access to AI developer skillsets, and it’s clear again with Lemurian’s strategy to redefine the mathematical foundations of AI and deliver more performant and efficient computing solutions.
With all the coverage on AI in 2023, one would need to be hiding from technology to not understand the size and urgency for demand of AI infrastructure. Today, however, AI training capabilities are locked within the largest of cloud providers due to limitations of access to the compute scale required to fuel training and the developer prowess to code AI effectively. The need for an alternative architecture is drawing the investor community to lean forward into AI silicon startups like Lemurian and customers to lean forward into consideration of alternative solutions.
Lemurian’s approach is rooted in silicon innovation but starts arguably even more foundationally in new math for AI. In our interview Jay described previous math models as great for silicon but not necessarily ideal for developers calling out INT8 as a prime example of an approach that challenged developer effectiveness.
Lemurian’s approach is rooted in logarithmic innovation. Jay explained, “We went back to a really old idea. Log number systems. It's the holy grail of number systems that if you can make a purely log link format work, and it's a 250 year old math problem. We came up with a way of actually making addition in a log format work, and we've developed multiple types, all of them, two bits to 64 bits, to stack up against floating point. So pound for pound or bit for bit, this thing has better precision, dynamic range and signal to noise ratio. This thing wins all day long.”
If you can re-map the number system that silicon uses to calculate, you can arguably achieve a Moore’s Law like increase in performance, something that AI sorely needs to break through with the compute efficiency required. In Jay’s presentation at the conference, he argued that the combination of accelerator silicon innovation, software improvement and number system advancement could yield as high as a 20X performance leap which explains why his sessions at the conference drew standing room crowds. He backed that up further showcasing eye opening simulations of 11,357 inferences a second for BERT Large and 119,278 inferences a second for ResNet50 based on a single node configuration. To put this in perspective, if these numbers hold they’ll beat NVIDIA’s A100 today which is what is widely deployed in the market.
So what is TechArena’s take? There are absolutely AI inferencing challenges at scale that require acceleration. Facebook’s workloads come to mind requiring nimble acceleration to meet latency requirements from users. While CPUs will remain the standard for inferencing performance efficiency, acceleration will continue to gain inroads for workloads that make sense opening a door for vendors like Lemurian. If Lemurian’s number system gains traction with developers the opportunity for the company will scale significantly, and this is where we’ll be watching most acutely as this exciting area of the industry progresses. For now, I love that Jay and team are challenging the status quo and stating emphatically that a tool as powerful as AI requires new ideas for democratic delivery. I’ll be watching what comes next from this exciting startup.
One thing you notice when you talk to Jay Dawani is that when he sees a challenge that’s important enough, he actually takes it on himself to solve it. This was made clear when Jay delivered the how to for coding AI algorithms based on a foundation of high school math to democratize access to AI developer skillsets, and it’s clear again with Lemurian’s strategy to redefine the mathematical foundations of AI and deliver more performant and efficient computing solutions.
With all the coverage on AI in 2023, one would need to be hiding from technology to not understand the size and urgency for demand of AI infrastructure. Today, however, AI training capabilities are locked within the largest of cloud providers due to limitations of access to the compute scale required to fuel training and the developer prowess to code AI effectively. The need for an alternative architecture is drawing the investor community to lean forward into AI silicon startups like Lemurian and customers to lean forward into consideration of alternative solutions.
Lemurian’s approach is rooted in silicon innovation but starts arguably even more foundationally in new math for AI. In our interview Jay described previous math models as great for silicon but not necessarily ideal for developers calling out INT8 as a prime example of an approach that challenged developer effectiveness.
Lemurian’s approach is rooted in logarithmic innovation. Jay explained, “We went back to a really old idea. Log number systems. It's the holy grail of number systems that if you can make a purely log link format work, and it's a 250 year old math problem. We came up with a way of actually making addition in a log format work, and we've developed multiple types, all of them, two bits to 64 bits, to stack up against floating point. So pound for pound or bit for bit, this thing has better precision, dynamic range and signal to noise ratio. This thing wins all day long.”
If you can re-map the number system that silicon uses to calculate, you can arguably achieve a Moore’s Law like increase in performance, something that AI sorely needs to break through with the compute efficiency required. In Jay’s presentation at the conference, he argued that the combination of accelerator silicon innovation, software improvement and number system advancement could yield as high as a 20X performance leap which explains why his sessions at the conference drew standing room crowds. He backed that up further showcasing eye opening simulations of 11,357 inferences a second for BERT Large and 119,278 inferences a second for ResNet50 based on a single node configuration. To put this in perspective, if these numbers hold they’ll beat NVIDIA’s A100 today which is what is widely deployed in the market.
So what is TechArena’s take? There are absolutely AI inferencing challenges at scale that require acceleration. Facebook’s workloads come to mind requiring nimble acceleration to meet latency requirements from users. While CPUs will remain the standard for inferencing performance efficiency, acceleration will continue to gain inroads for workloads that make sense opening a door for vendors like Lemurian. If Lemurian’s number system gains traction with developers the opportunity for the company will scale significantly, and this is where we’ll be watching most acutely as this exciting area of the industry progresses. For now, I love that Jay and team are challenging the status quo and stating emphatically that a tool as powerful as AI requires new ideas for democratic delivery. I’ll be watching what comes next from this exciting startup.