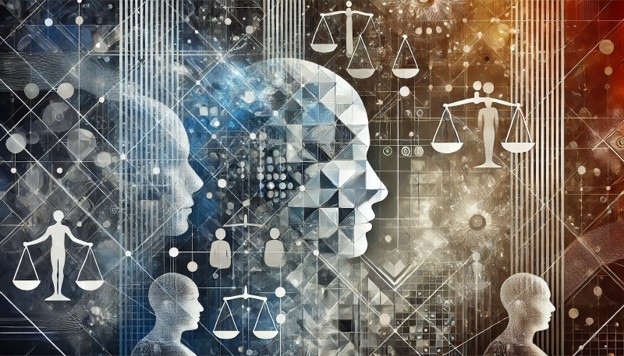
Digital Ethics in AI: 3 Key Considerations for Leaders
In the last year, we’ve seen significant growth in the adoption of artificial intelligence (AI). One way to judge a technology’s maturity is to find its place on the Gartner Hype cycle. The challenge here is that “AI” is an umbrella name for a variety of distinct AI systems. And thus, there is no one place where we can put AI, but rather AI deserves multiple entries in the hype scale to demonstrate its true impact according to this measure.
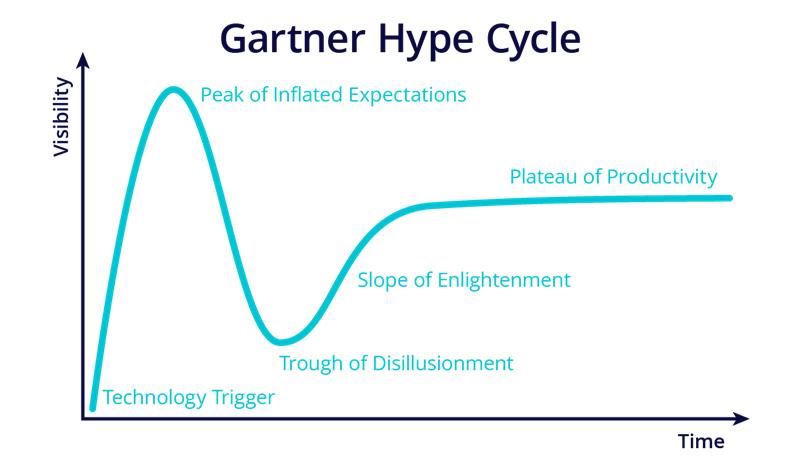
Expert systems and narrow AI are more mature as they move into the plateau of productivity. But one of the more familiar systems, Generative AI, seems to have moved beyond the Peak of Inflated Expectations into a phase where companies are grappling with practical implementation challenges like ethical concerns, computational demands and sustainability challenges.
While this big technological revolution has enormous potential, implementing AI comes with its own set of unique challenges. We’ve all seen some of the more public examples of what can happen when AI gets it wrong – including recent instances of AI applications showing bias. This is where businesses need to de-risk the implementation of AI and ensure AI systems can be a force multiplier for the business rather than a counterproductive or disruptive element.
From an ethical perspective I believe there are three high level considerations for organizations determining their AI strategies.
- Bias & Fairness
The first thing is to have a good awareness and understanding of the system you are implementing and WHY. It’s important that this awareness and understanding spans your organization from procurement to senior leadership so all involved in the decision process can ask the right questions. Many free resources exist to get educated on the strengths and weaknesses of AI technology. This technology is rapidly evolving, so it is important to stay informed about the status quo.
Without awareness and understanding, we focus on the promise but overlook the implications. While you could potentially have significant gains in productivity, the flip side is that your AI system may be generating unfair outcomes because it’s biased and/or is discriminating. I’ve seen it firsthand when a client I was working with experimented with a vision-based recognition system for advertisement that didn’t work for people from certain demographics.
2. Transparency & Explainability
The other part of this is the WHAT. Be very clear on WHAT you want to achieve by implementing the technology and what benefit you want to realize. Once that is clear, de-risk the ideal outcome. Per example: If you implement a computer vision system, what happens when an image is identified with a false-negative or positive? When you use a supervised learning system, what are the label criteria and how are they applied? Or what if your NLP system gets the translation wrong ever so slightly? What could the worst impact be? How do you ensure the quality of your answer? And if it is not accurate, how do you inform your audience of this possibility – very much like that sticker on your car mirror?

AI is a transformative and very powerful technology, but it’s also a black box technology – meaning its decision criteria and decision-making process are very hard to reverse engineer to verify and ensure accountability. Some good news here is that developments on XAI (explainable AI) are underway and rapidly evolving too. Another related research area of interest is machine unlearning, which will help further refine models when old, illegal or incorrect data need to be removed from existing models. Several universities and big tech companies are researching this capability. Both examples underline my initial point to keep abreast of the latest developments.
3. Privacy & Data Security
AI, by nature, requires large amounts of data to train and improve its models. Frequently, this can include private and personal data, which is a concern for how data is collected, stored and processed. And while regulations such as GDPR are put in place, the pace of AI innovations is already challenging these legal frameworks in areas like data minimization, user consent and decision making. Per example: AI algorithms could leverage personal data in ways that weren’t foreseen when collecting this information, making it very challenging to get informed, and specific user consent, that GDPR requires.
Methods to address these challenges include data minimization and anonymization techniques and informing users that the data they submit for collection and processing can be used for AI purposes. As stated before, this is complex to implement, but certainly something to keep front-and-center when discussing AI.
Awareness and Education
Implementing AI requires continuous education and awareness. This is critical as this field and its capabilities are ever expanding. With the arrival of Generative AI, there has been mass adoption, but it has not been accompanied by mass education. This is a gap that will need to be addressed over time. Education moves slowly, but it has big impact in the long haul.
Driving more awareness of AI capabilities is at an all-time high as AI is right on top of the hype cycle and projections of economic gains in productivity are skyrocketing. This drives natural interest and hype from the industry. But awareness for some of the limitations and impact of AI and some of the ethical solutions is low and it’s up to us in the industry to share the learnings.
One of the best examples to date that I’ve seen is this highly educational YouTube video by Andrej Karpathy who is an AI researcher who worked at OpenAI and Tesla. Not only does he explain and provide examples of how Large Language models (LLMs) work, but he explains the security risks of LLMs (timestamp 45:44) and how prompt injection, data poisoning and jailbreaks can affect LLMs. He provides examples for these scenarios, too. The industry needs more of this kind of content and educational leadership.
There is more to be optimistic and encouraged by. I recently attended the Worldsummit.AI event in Amsterdam. And besides real-world implementation examples, it had dedicated tracks for responsible AI and governance and HUMAN-AI convergence and created a platform to discuss the current and future impact of AI on society. It’s clear that the democratization of AI comes with lots of benefits, but it also includes a worrying factor. Next to all the technological limitations, there are also the malicious actors who will continue to exploit this technology, which adds to the complexity of managing it all. Legislation will help mitigate some of the negative impacts.
Walking away from these two intense days, I’d like to share some observations from my discussions:
The first one is, “Every company is an AI company,” whether they have a strategy or not. Employees are experimenting and implementing.
The second one is that AI risk assessments seem to be the biggest driver of use-case adoption in the industry now. This assessment is typically done by an independent third party. And it seems to be a good balance between innovation and (to a degree) ethical implementation. It puts tension in the system on the checks and balances of outcomes and impact.
However, as one panelist discussing third party validation of AI mentioned, “Some things are checkable; some things are not...”
In summary
As with any technology, it’s critical to keep an open, curious and objective mind when thinking of AI applications and ensure due diligence on the digital ethical aspects of the implementation. We are all students of this new technology, so it’s on us to put the right guard rails in place.
Get Involved
If this topic holds your interest, I’d love for you to join the D.E.A.P. (digital ethics awareness platform) LinkedIn group. I’ve recently created it with the intent of bringing more awareness to the capabilities and ethical solutions and research available. This way, we can all make better informed decisions. Feel free to join and contribute relevant information here: https://www.linkedin.com/groups/13079342/.
In the last year, we’ve seen significant growth in the adoption of artificial intelligence (AI). One way to judge a technology’s maturity is to find its place on the Gartner Hype cycle. The challenge here is that “AI” is an umbrella name for a variety of distinct AI systems. And thus, there is no one place where we can put AI, but rather AI deserves multiple entries in the hype scale to demonstrate its true impact according to this measure.
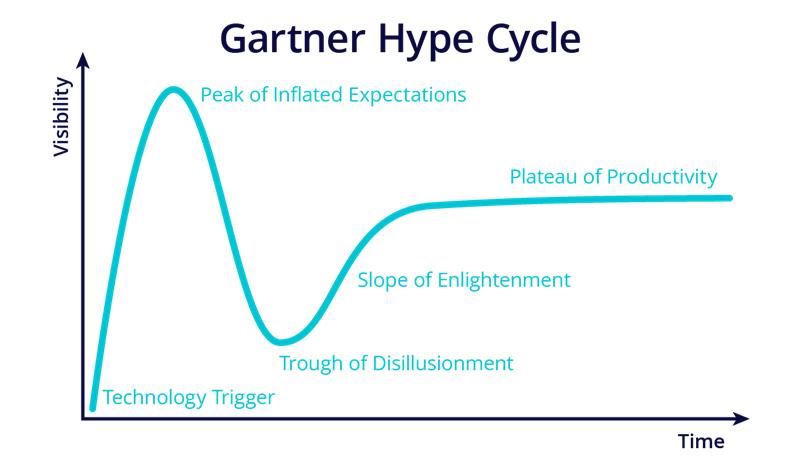
Expert systems and narrow AI are more mature as they move into the plateau of productivity. But one of the more familiar systems, Generative AI, seems to have moved beyond the Peak of Inflated Expectations into a phase where companies are grappling with practical implementation challenges like ethical concerns, computational demands and sustainability challenges.
While this big technological revolution has enormous potential, implementing AI comes with its own set of unique challenges. We’ve all seen some of the more public examples of what can happen when AI gets it wrong – including recent instances of AI applications showing bias. This is where businesses need to de-risk the implementation of AI and ensure AI systems can be a force multiplier for the business rather than a counterproductive or disruptive element.
From an ethical perspective I believe there are three high level considerations for organizations determining their AI strategies.
- Bias & Fairness
The first thing is to have a good awareness and understanding of the system you are implementing and WHY. It’s important that this awareness and understanding spans your organization from procurement to senior leadership so all involved in the decision process can ask the right questions. Many free resources exist to get educated on the strengths and weaknesses of AI technology. This technology is rapidly evolving, so it is important to stay informed about the status quo.
Without awareness and understanding, we focus on the promise but overlook the implications. While you could potentially have significant gains in productivity, the flip side is that your AI system may be generating unfair outcomes because it’s biased and/or is discriminating. I’ve seen it firsthand when a client I was working with experimented with a vision-based recognition system for advertisement that didn’t work for people from certain demographics.
2. Transparency & Explainability
The other part of this is the WHAT. Be very clear on WHAT you want to achieve by implementing the technology and what benefit you want to realize. Once that is clear, de-risk the ideal outcome. Per example: If you implement a computer vision system, what happens when an image is identified with a false-negative or positive? When you use a supervised learning system, what are the label criteria and how are they applied? Or what if your NLP system gets the translation wrong ever so slightly? What could the worst impact be? How do you ensure the quality of your answer? And if it is not accurate, how do you inform your audience of this possibility – very much like that sticker on your car mirror?

AI is a transformative and very powerful technology, but it’s also a black box technology – meaning its decision criteria and decision-making process are very hard to reverse engineer to verify and ensure accountability. Some good news here is that developments on XAI (explainable AI) are underway and rapidly evolving too. Another related research area of interest is machine unlearning, which will help further refine models when old, illegal or incorrect data need to be removed from existing models. Several universities and big tech companies are researching this capability. Both examples underline my initial point to keep abreast of the latest developments.
3. Privacy & Data Security
AI, by nature, requires large amounts of data to train and improve its models. Frequently, this can include private and personal data, which is a concern for how data is collected, stored and processed. And while regulations such as GDPR are put in place, the pace of AI innovations is already challenging these legal frameworks in areas like data minimization, user consent and decision making. Per example: AI algorithms could leverage personal data in ways that weren’t foreseen when collecting this information, making it very challenging to get informed, and specific user consent, that GDPR requires.
Methods to address these challenges include data minimization and anonymization techniques and informing users that the data they submit for collection and processing can be used for AI purposes. As stated before, this is complex to implement, but certainly something to keep front-and-center when discussing AI.
Awareness and Education
Implementing AI requires continuous education and awareness. This is critical as this field and its capabilities are ever expanding. With the arrival of Generative AI, there has been mass adoption, but it has not been accompanied by mass education. This is a gap that will need to be addressed over time. Education moves slowly, but it has big impact in the long haul.
Driving more awareness of AI capabilities is at an all-time high as AI is right on top of the hype cycle and projections of economic gains in productivity are skyrocketing. This drives natural interest and hype from the industry. But awareness for some of the limitations and impact of AI and some of the ethical solutions is low and it’s up to us in the industry to share the learnings.
One of the best examples to date that I’ve seen is this highly educational YouTube video by Andrej Karpathy who is an AI researcher who worked at OpenAI and Tesla. Not only does he explain and provide examples of how Large Language models (LLMs) work, but he explains the security risks of LLMs (timestamp 45:44) and how prompt injection, data poisoning and jailbreaks can affect LLMs. He provides examples for these scenarios, too. The industry needs more of this kind of content and educational leadership.
There is more to be optimistic and encouraged by. I recently attended the Worldsummit.AI event in Amsterdam. And besides real-world implementation examples, it had dedicated tracks for responsible AI and governance and HUMAN-AI convergence and created a platform to discuss the current and future impact of AI on society. It’s clear that the democratization of AI comes with lots of benefits, but it also includes a worrying factor. Next to all the technological limitations, there are also the malicious actors who will continue to exploit this technology, which adds to the complexity of managing it all. Legislation will help mitigate some of the negative impacts.
Walking away from these two intense days, I’d like to share some observations from my discussions:
The first one is, “Every company is an AI company,” whether they have a strategy or not. Employees are experimenting and implementing.
The second one is that AI risk assessments seem to be the biggest driver of use-case adoption in the industry now. This assessment is typically done by an independent third party. And it seems to be a good balance between innovation and (to a degree) ethical implementation. It puts tension in the system on the checks and balances of outcomes and impact.
However, as one panelist discussing third party validation of AI mentioned, “Some things are checkable; some things are not...”
In summary
As with any technology, it’s critical to keep an open, curious and objective mind when thinking of AI applications and ensure due diligence on the digital ethical aspects of the implementation. We are all students of this new technology, so it’s on us to put the right guard rails in place.
Get Involved
If this topic holds your interest, I’d love for you to join the D.E.A.P. (digital ethics awareness platform) LinkedIn group. I’ve recently created it with the intent of bringing more awareness to the capabilities and ethical solutions and research available. This way, we can all make better informed decisions. Feel free to join and contribute relevant information here: https://www.linkedin.com/groups/13079342/.