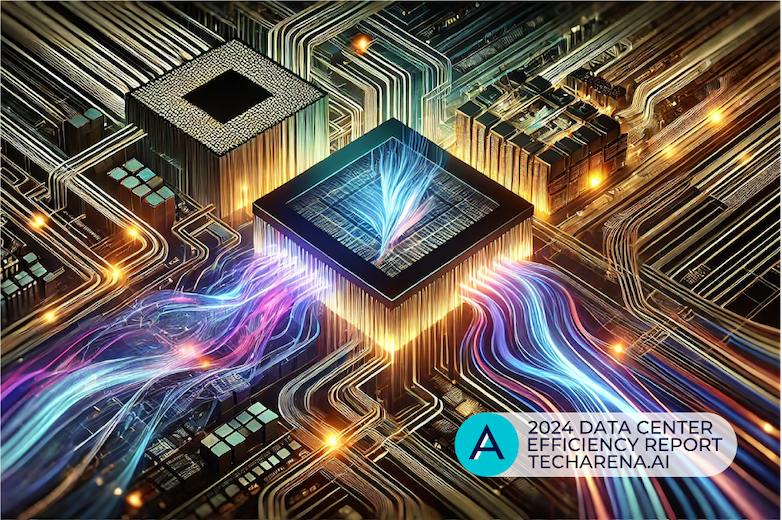
5 Fast Facts with Justin Murrill at AMD
I recently caught up with Justin Murrill at AMD to learn more about how processor innovation helps yield improved efficiency to data center workload delivery. AMD is delivering leading compute silicon with a broad offering of foundational CPU and acceleration technologies aimed at data center environments.
Justin is the AMD Director of Corporate Responsibility and is responsible for coordinating the company's sustainability strategy, goals and reporting.
ALLYSON: Justin, thank you for being here today. As you know, AI has fundamentally changed the requirements for computing in the data center. With estimates that >40% of all servers will be dedicated to AI by 2026, how is AMD seeing computing evolving across CPU, GPU, and FPGA?
JUSTIN: As AI continues to evolve rapidly, having a broad range of compute engines will be critical to scaling performance and efficiency. We see each of these different compute engines playing a unique role, particularly as AI workloads shift and as use models evolve. We also look to enable more on-device processing across the edge and end devices — further helping to drive efficiencies. Having our full range of processing engines allows customers to balance the competing demands for disparate performance levels, throughput, capacity, energy consumption, programmability and available space. Offering compute engines that address these needs while increasingly accommodating AI requirements is a critical differentiator for AMD.
ALLYSON: This increased compute demand is placing greater emphasis on compute efficiency. How does AMD integrate efficient design into roadmap planning, and what has this influenced in your architecture?
JUSTIN: The computing performance delivered per watt of energy consumed is a vital aspect of our business strategy. Our products’ cutting-edge chip architecture, design, and power management features have resulted in significant energy efficiency gains. AMD has a track record of setting long-term energy efficiency goals and incorporating them into engineering roadmaps for successful execution.
We have a bold goal to achieve a 30x improvement in energy efficiency for AMD processors and accelerators powering HPC and AI training by 2025—our “30x25 goal”.1 If all AI and HPC server nodes globally were to make similar gains to the AMD 30x25 goal, we estimate billions of kilowatt-hours of electricity could be saved in 2025, relative to baseline trends.2 Achieving the goal would also mean we would exceed the industry trendline for energy efficiency gains and reduce energy use per computation by up to 97% as compared to 2020. As of late 2023, we achieved a 13.5x improvement in energy efficiency from the 2020 baseline, using a configuration of four AMD Instinct MI300A APUs (4th Gen AMD EPYC™ CPU with AMD CDNA™ 3 Compute Units).3 Similarly, this kind of philosophy and process helped influence design choices across our “Zen” architectures for the AMD EPYC server CPU family—to develop a core infrastructure that balances performance, efficiency and density.
To embed energy efficiency goals into the design process, we collaborate closely with customers to understand their unique needs for performance, power targets, features and more. All this input is included in the design process, and teams have different stages in the process to check on the alignment with the end functionality that they are targeting.
One example of how AMD is addressing customer performance needs and configuration flexibility, while also advancing sustainability, is the modular architecture we pioneered with chiplets. Instead of one large monolithic chip, AMD engineers reconfigured the component IP building blocks using a flexible, scalable connectivity we designed known as Infinity Fabric. This laid the foundation for our Infinity Architecture, which enables us to configure multiple individual chiplets to scale compute cores in countless designs. Not only does this further optimize energy efficiency, but it also reduces environmental impacts in the wafer manufacturing process.
By breaking our designs up into smaller chiplets, we can get more chips per wafer, lowering the probability that a defect will land on any one chip. As a result, the number and yield percentage of “good” chips per wafer goes up, and the wasted cost, raw materials, energy, emissions, and water goes down. For example, producing 4th Gen AMD EPYC™ CPUs with up to 12 separate compute chiplets instead of one monolithic die saved approximately 132,000 metric tons of CO2e in 2023 through avoidance of wafers manufactured, 2.8 times more than the annual operational CO2e footprint of AMD in 2023.4
ALLYSON: With markets like the EU placing greater emphasis on embedded carbon and other sustainability metrics, how is AMD working with manufacturing partners to deliver transparency within your product offerings?
JUSTIN: We work with our Manufacturing Suppliers to advance environmental sustainability across a variety of metrics, including emissions related to AMD purchased goods and services (Scope 3 emissions). Aligned with guidance from the GHG Protocol, we directly survey manufacturing suppliers representing ~95% of our related supply chain spend and use the data to estimate and publicly report our related Scope 3 emissions.
We also set goals for our manufacturing suppliers and report our progress annually. Our 2025 public goals are for 100% of manufacturing suppliers to have their own public GHG reduction goal(s) and 80% to source renewable energy. Our latest report shows 84% of our Manufacturing Suppliers had public GHG goals and 71% source renewable energy.
Carbon emissions in our supply chain are primarily generated at silicon wafer manufacturing facilities. Most AMD wafers come from TSMC, which implemented more than 800 energy savings measures in 2023, saving approximately 92,960 MWh of energy and 46,000 metric tons of CO2e attributed to AMD wafer production. In September 2023, TSMC announced an accelerated renewable energy roadmap, increasing its 2030 target from 40% to 60% of the total energy supply, and pulling in its 100% renewable energy target from 2050 to 2040.
In the near term, the amount of renewable energy available in Taiwan and other regions in Asia is very limited. To help address this challenge, AMD is a founding member of the Semiconductor Climate Consortium and a sponsor of its Energy Collaborative working to accelerate renewable energy access in the Asia-Pacific region.
ALLYSON: How do you see AI actually influencing the delivery of efficient compute as a strategic tool for AMD innovation in the future?
JUSTIN: Energy efficiency has always been a key pillar of our design and ecosystem enablement approach, and we take a holistic view at the silicon, software and system level to get the most performance and functionality out of every watt consumed. The benefits are so much more impactful when hardware, software and systems can evolve in more relative lockstep, which requires deep technical collaborations.
We also embrace the scaling of efficiency impacts that AI can enable as it matures and proliferates – from helping assign compute resources more optimally to monitoring and diagnosing underperforming hardware. Some of the leading application vendors we collaborate with in electronic design, simulation and verification are developing AI-enabled tools to optimize system and cluster performance, automate and augment common routines, reduce waste and improve productivity.
ALLYSON: Where can our readers find out more about AMD solutions in this space and engage the AMD team to learn more?
JUSTIN:
- Explore the AMD 2023-24 Corporate Responsibility Report
- Learn more about how AMD is advancing data center sustainability here
- Get the details on AMD EPYC server CPU energy efficiency here
- Use the AMD TCO Greenhouse Gas Calculators – Bare Metal and Virtualization versions
1 Includes AMD high-performance CPU and GPU accelerators used for AI-training and high-performance computing in a 4-Accelerator, CPU-hosted configuration. Goal calculations are based on performance scores as measured by standard performance metrics (HPC: Linpack DGEMM kernel FLOPS with 4k matrix size; AI-training: lower precision training-focused floating-point math GEMM kernels such as FP16 or BF16 FLOPS operating on 4k matrices) divided by the rated power consumption of a representative accelerated compute node, including the CPU host + memory and 4 GPU accelerators.
2 “Data Center Sustainability,” AMD, https://www.amd.com/en/corporate/corporate-responsibility/data-center-sustainability.html (accessed May 15, 2024).
3 EPYC-030a: Calculation includes 1) base case kWhr use projections in 2025 conducted with Koomey Analytics based on available research and data that includes segment specific projected 2025 deployment volumes and data center power utilization effectiveness (PUE) including GPU HPC and machine learning (ML) installations and 2) AMD CPU and GPU node power consumptions incorporating segment-specific utilization (active vs. idle) percentages and multiplied by PUE to determine actual total energy use for calculation of the performance per Watt. 13.5x is calculated using the following formula: (base case HPC node kWhr use projection in 2025 * AMD 2023 perf/Watt improvement using DGEMM and TEC +Base case ML node kWhr use projection in 2025 *AMD 2023 perf/Watt improvement using ML math and TEC) /(2020 perf/Watt * Base case projected kWhr usage in 2025). For more information, www.amd.com/en/corporate-responsibility/data-center-sustainability
4 AMD estimation based on defect density (defects per unit area on the wafer), chip area and n-factor (manufacturing complexity factor) to estimate the number of wafers avoided in one year. Yield = (1 + A*D0)^(-n) where A is the chip area, D0 is the defect density and n is the complexity factor. The area is known from our design, D0 is known based our manufacturing yield data, and n is a number provided by a foundry partner for a given technology. The calculations are not meant to be precise, since chip design can have a large influence on yield, but it estimates the area impact on yield. The carbon emission estimates of 132,064 mtCO2e were calculated using the estimated number of 5 nm wafers saved in one year, based on the TechInsights’ Semiconductor Manufacturing Carbon Model. Comparison to AMD corporate footprint is based on AMD reported scope 1 and 2 market-based GHG emissions in 2023: 46,606 mtCO2e. Water savings estimates of 1,110 million liters were calculated using the estimated number of 5 nm wafers saved in one year times the amount of water use per 300mm wafer mask layer times the average number of mask layers. Comparison to AMD corporate water use is based on AMD 2023 reported value of 225 million liters.
I recently caught up with Justin Murrill at AMD to learn more about how processor innovation helps yield improved efficiency to data center workload delivery. AMD is delivering leading compute silicon with a broad offering of foundational CPU and acceleration technologies aimed at data center environments.
Justin is the AMD Director of Corporate Responsibility and is responsible for coordinating the company's sustainability strategy, goals and reporting.
ALLYSON: Justin, thank you for being here today. As you know, AI has fundamentally changed the requirements for computing in the data center. With estimates that >40% of all servers will be dedicated to AI by 2026, how is AMD seeing computing evolving across CPU, GPU, and FPGA?
JUSTIN: As AI continues to evolve rapidly, having a broad range of compute engines will be critical to scaling performance and efficiency. We see each of these different compute engines playing a unique role, particularly as AI workloads shift and as use models evolve. We also look to enable more on-device processing across the edge and end devices — further helping to drive efficiencies. Having our full range of processing engines allows customers to balance the competing demands for disparate performance levels, throughput, capacity, energy consumption, programmability and available space. Offering compute engines that address these needs while increasingly accommodating AI requirements is a critical differentiator for AMD.
ALLYSON: This increased compute demand is placing greater emphasis on compute efficiency. How does AMD integrate efficient design into roadmap planning, and what has this influenced in your architecture?
JUSTIN: The computing performance delivered per watt of energy consumed is a vital aspect of our business strategy. Our products’ cutting-edge chip architecture, design, and power management features have resulted in significant energy efficiency gains. AMD has a track record of setting long-term energy efficiency goals and incorporating them into engineering roadmaps for successful execution.
We have a bold goal to achieve a 30x improvement in energy efficiency for AMD processors and accelerators powering HPC and AI training by 2025—our “30x25 goal”.1 If all AI and HPC server nodes globally were to make similar gains to the AMD 30x25 goal, we estimate billions of kilowatt-hours of electricity could be saved in 2025, relative to baseline trends.2 Achieving the goal would also mean we would exceed the industry trendline for energy efficiency gains and reduce energy use per computation by up to 97% as compared to 2020. As of late 2023, we achieved a 13.5x improvement in energy efficiency from the 2020 baseline, using a configuration of four AMD Instinct MI300A APUs (4th Gen AMD EPYC™ CPU with AMD CDNA™ 3 Compute Units).3 Similarly, this kind of philosophy and process helped influence design choices across our “Zen” architectures for the AMD EPYC server CPU family—to develop a core infrastructure that balances performance, efficiency and density.
To embed energy efficiency goals into the design process, we collaborate closely with customers to understand their unique needs for performance, power targets, features and more. All this input is included in the design process, and teams have different stages in the process to check on the alignment with the end functionality that they are targeting.
One example of how AMD is addressing customer performance needs and configuration flexibility, while also advancing sustainability, is the modular architecture we pioneered with chiplets. Instead of one large monolithic chip, AMD engineers reconfigured the component IP building blocks using a flexible, scalable connectivity we designed known as Infinity Fabric. This laid the foundation for our Infinity Architecture, which enables us to configure multiple individual chiplets to scale compute cores in countless designs. Not only does this further optimize energy efficiency, but it also reduces environmental impacts in the wafer manufacturing process.
By breaking our designs up into smaller chiplets, we can get more chips per wafer, lowering the probability that a defect will land on any one chip. As a result, the number and yield percentage of “good” chips per wafer goes up, and the wasted cost, raw materials, energy, emissions, and water goes down. For example, producing 4th Gen AMD EPYC™ CPUs with up to 12 separate compute chiplets instead of one monolithic die saved approximately 132,000 metric tons of CO2e in 2023 through avoidance of wafers manufactured, 2.8 times more than the annual operational CO2e footprint of AMD in 2023.4
ALLYSON: With markets like the EU placing greater emphasis on embedded carbon and other sustainability metrics, how is AMD working with manufacturing partners to deliver transparency within your product offerings?
JUSTIN: We work with our Manufacturing Suppliers to advance environmental sustainability across a variety of metrics, including emissions related to AMD purchased goods and services (Scope 3 emissions). Aligned with guidance from the GHG Protocol, we directly survey manufacturing suppliers representing ~95% of our related supply chain spend and use the data to estimate and publicly report our related Scope 3 emissions.
We also set goals for our manufacturing suppliers and report our progress annually. Our 2025 public goals are for 100% of manufacturing suppliers to have their own public GHG reduction goal(s) and 80% to source renewable energy. Our latest report shows 84% of our Manufacturing Suppliers had public GHG goals and 71% source renewable energy.
Carbon emissions in our supply chain are primarily generated at silicon wafer manufacturing facilities. Most AMD wafers come from TSMC, which implemented more than 800 energy savings measures in 2023, saving approximately 92,960 MWh of energy and 46,000 metric tons of CO2e attributed to AMD wafer production. In September 2023, TSMC announced an accelerated renewable energy roadmap, increasing its 2030 target from 40% to 60% of the total energy supply, and pulling in its 100% renewable energy target from 2050 to 2040.
In the near term, the amount of renewable energy available in Taiwan and other regions in Asia is very limited. To help address this challenge, AMD is a founding member of the Semiconductor Climate Consortium and a sponsor of its Energy Collaborative working to accelerate renewable energy access in the Asia-Pacific region.
ALLYSON: How do you see AI actually influencing the delivery of efficient compute as a strategic tool for AMD innovation in the future?
JUSTIN: Energy efficiency has always been a key pillar of our design and ecosystem enablement approach, and we take a holistic view at the silicon, software and system level to get the most performance and functionality out of every watt consumed. The benefits are so much more impactful when hardware, software and systems can evolve in more relative lockstep, which requires deep technical collaborations.
We also embrace the scaling of efficiency impacts that AI can enable as it matures and proliferates – from helping assign compute resources more optimally to monitoring and diagnosing underperforming hardware. Some of the leading application vendors we collaborate with in electronic design, simulation and verification are developing AI-enabled tools to optimize system and cluster performance, automate and augment common routines, reduce waste and improve productivity.
ALLYSON: Where can our readers find out more about AMD solutions in this space and engage the AMD team to learn more?
JUSTIN:
- Explore the AMD 2023-24 Corporate Responsibility Report
- Learn more about how AMD is advancing data center sustainability here
- Get the details on AMD EPYC server CPU energy efficiency here
- Use the AMD TCO Greenhouse Gas Calculators – Bare Metal and Virtualization versions
1 Includes AMD high-performance CPU and GPU accelerators used for AI-training and high-performance computing in a 4-Accelerator, CPU-hosted configuration. Goal calculations are based on performance scores as measured by standard performance metrics (HPC: Linpack DGEMM kernel FLOPS with 4k matrix size; AI-training: lower precision training-focused floating-point math GEMM kernels such as FP16 or BF16 FLOPS operating on 4k matrices) divided by the rated power consumption of a representative accelerated compute node, including the CPU host + memory and 4 GPU accelerators.
2 “Data Center Sustainability,” AMD, https://www.amd.com/en/corporate/corporate-responsibility/data-center-sustainability.html (accessed May 15, 2024).
3 EPYC-030a: Calculation includes 1) base case kWhr use projections in 2025 conducted with Koomey Analytics based on available research and data that includes segment specific projected 2025 deployment volumes and data center power utilization effectiveness (PUE) including GPU HPC and machine learning (ML) installations and 2) AMD CPU and GPU node power consumptions incorporating segment-specific utilization (active vs. idle) percentages and multiplied by PUE to determine actual total energy use for calculation of the performance per Watt. 13.5x is calculated using the following formula: (base case HPC node kWhr use projection in 2025 * AMD 2023 perf/Watt improvement using DGEMM and TEC +Base case ML node kWhr use projection in 2025 *AMD 2023 perf/Watt improvement using ML math and TEC) /(2020 perf/Watt * Base case projected kWhr usage in 2025). For more information, www.amd.com/en/corporate-responsibility/data-center-sustainability
4 AMD estimation based on defect density (defects per unit area on the wafer), chip area and n-factor (manufacturing complexity factor) to estimate the number of wafers avoided in one year. Yield = (1 + A*D0)^(-n) where A is the chip area, D0 is the defect density and n is the complexity factor. The area is known from our design, D0 is known based our manufacturing yield data, and n is a number provided by a foundry partner for a given technology. The calculations are not meant to be precise, since chip design can have a large influence on yield, but it estimates the area impact on yield. The carbon emission estimates of 132,064 mtCO2e were calculated using the estimated number of 5 nm wafers saved in one year, based on the TechInsights’ Semiconductor Manufacturing Carbon Model. Comparison to AMD corporate footprint is based on AMD reported scope 1 and 2 market-based GHG emissions in 2023: 46,606 mtCO2e. Water savings estimates of 1,110 million liters were calculated using the estimated number of 5 nm wafers saved in one year times the amount of water use per 300mm wafer mask layer times the average number of mask layers. Comparison to AMD corporate water use is based on AMD 2023 reported value of 225 million liters.